- Advertising
- Bare Metal
- Bare Metal Cloud
- Benchmarks
- Big Data Benchmarks
- Big Data Experts Interviews
- Big Data Technologies
- Big Data Use Cases
- Big Data Week
- Cloud
- Data Lake as a Service
- Databases
- Dedicated Servers
- Disaster Recovery
- Features
- Fun
- GoTech World
- Hadoop
- Healthcare
- Industry Standards
- Insurance
- Linux
- News
- NoSQL
- Online Retail
- People of Bigstep
- Performance for Big Data Apps
- Press
- Press Corner
- Security
- Tech Trends
- Tutorial
- What is Big Data
Three Ways You're Getting It All Wrong With Big Data
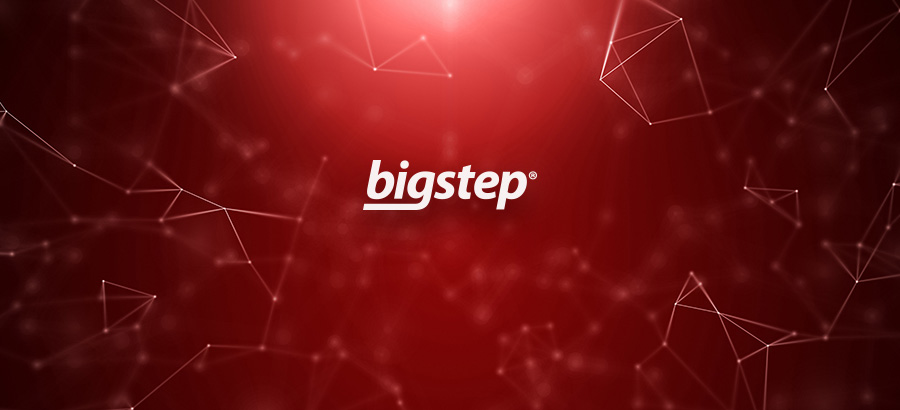
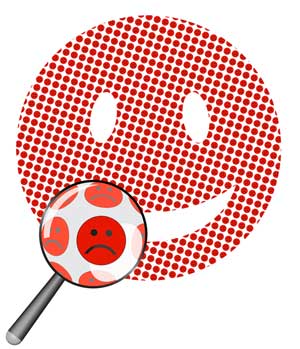
Don’t you hate it when some smart aleck tells you you’re doing it wrong?
In the case of big data, the potential for how you utilize it is so great there’s always room for improvement.
But before you take the next giant leap toward big data innovation, you must level the playing field by recognizing some common pitfalls leading organizations astray. Understanding baseline best practices in big data analytics include focusing on where it originates and how you parse it.
Big Data Analytics Pitfalls
Correlation ≠ Causation
All data isn’t equal, of course, so beware of assuming correlation equals causation. One infamous example is researchers suggesting childhood vaccinations cause autism. Health providers have widely discarded this conclusion. Taking a causal deeper dive instead of settling for surface correlation is a challenge. Yet correlation is the equivalent of circumstantial evidence in a court of law. While the outcome may appear a no-brainer, courts still strive to establish causation—with mixed results. Generating incorrect conclusions from correlating data can be just as damaging to your organization.
Data Bias
Are you filtering data to fit your desired hypothesis? While this commonly occurs in politics, your enterprise should avoid it. Information Week has an article outlining seven typical data biases in the business world, and it makes for an informative (and sometimes disheartening) read. The article points out common data biases that are some of the easiest mistakes to make. A common scenario includes a CEO requesting a department-level report subtly reinforcing a particular business strategy. However, we know the data must drive business decisions - not the other way around.
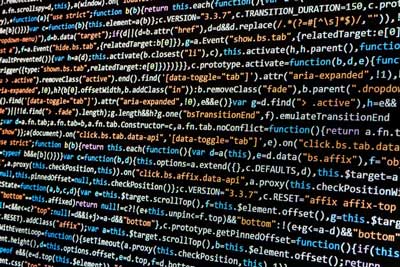
Data Falsification
In late 2016, social media picked up on the concept of fake news sources. Obviously, when we’re talking about data, the concept of “fake it till you make it” does not apply. Information Week suggests fake data is a growing problem in academic research. They suggest watching for a number of red flags that could signal the data lacks accuracy, including:
- Data hoarding, when one person is in control of the data and they work to deflect scrutiny of the methodology behind how they collect, store, or collate.
- Disorganized data that creates a “squirrel” moment, in order to distract from data scrutiny.
- When the results are simply too good to be true.
Data Rules
Even carpenters recommend measuring twice and cutting once. Try to apply the same rule to data, by running the metrics in different ways to ensure a more accurate outcome. This may sound like a no-brainer, but when you combine human inconsistencies in work ethics and skill sets across a large disparate enterprise, you’ll need to reestablish the most basic of data best practices. Failing to establish a baseline for data capture and dissemination across an organization is a major problem.
Bigstep combines big data apps with high-performance cloud computing. Our mission is to create, launch and scale big data that will enable business intelligence. Learn more about us and gather more tips on how you can avoid data pitfalls in the future.
Readers also enjoyed:
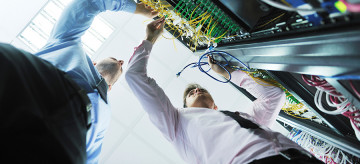
HP
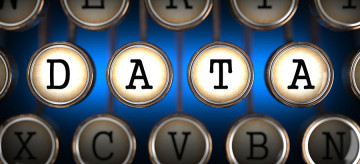
Leave a Reply
Your email address will not be published.