- Advertising
- Bare Metal
- Bare Metal Cloud
- Benchmarks
- Big Data Benchmarks
- Big Data Experts Interviews
- Big Data Technologies
- Big Data Use Cases
- Big Data Week
- Cloud
- Data Lake as a Service
- Databases
- Dedicated Servers
- Disaster Recovery
- Features
- Fun
- GoTech World
- Hadoop
- Healthcare
- Industry Standards
- Insurance
- Linux
- News
- NoSQL
- Online Retail
- People of Bigstep
- Performance for Big Data Apps
- Press
- Press Corner
- Security
- Tech Trends
- Tutorial
- What is Big Data
Calling the McLaren Team
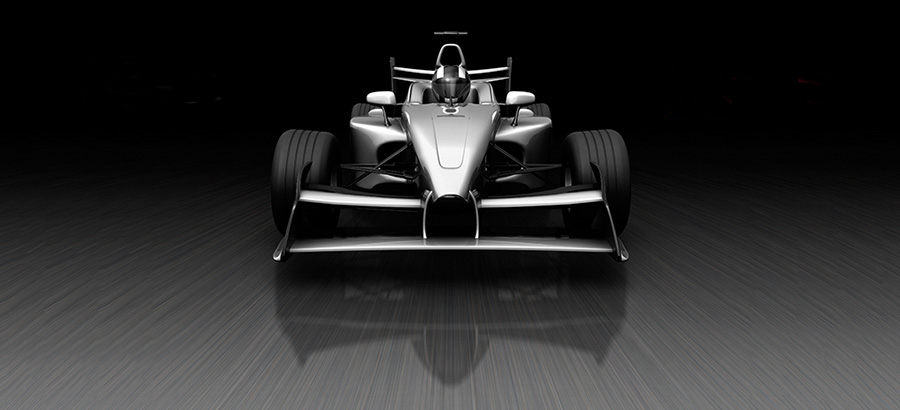
A team works to improve performance in Formula 1 racing. A couple of years later, they’re asked to help with airport efficiency, oil drilling and drug testing. Why?
It’s all because of big data. The McLaren Applied Technologiesteam, whose sole purpose was to run simulations and provide better solutions for the company’s racing team, has become famous during the 2008 Monaco Grand Prix. Their driver’s car, Lewis Hamilton, was in the second place when an accident occurred. It wasn’t life-threatening, but the car lost its right rear tire and Hamilton’s chances to regain the previous position diminished drastically. In only 6 seconds, the MAT team, who had been running real-time simulations and generating predictions after each lap, decided on a strategy. They took into account not only the car’s status, but also the fact that it was raining - a light shower that was about to end pretty soon. So they decided two things: they would fit the car with intermediate tires that would work equally well on wet and dry tracks; and they would add extra fuel. Both these decisions represented calculated risks. If the rain didn’t stop, the tires would be a disadvantage. The extra fuel added a lot of weight to the car - again, a disadvantage.
But their predictions payed off. The rain stopped, and as the other drivers pulled over to change their tires and refuel, Hamilton’s car was on track, steadily recovering. After 10 laps, he was in third place. When the last two drivers ahead of him had to pit, the extra fuel helped Hamilton remain on track and win the race.
Numbers and Cars and Racing Sports
Mark Williams, head of vehicle engineering, says it’s all probabilistic. The system looks at all the data and generates the most appropriate scenarios. That doesn’t make it perfect, but it gives the pilot the best possible chance to win.
The MAT team wanted to be efficient. Their thinking was to prepare the pilots for weeks before the race in a simulator and then, using all the data from there, apply it on the real thing in real-time. This proved to be a successful model. At first, pilots complained about the low quality of the graphics, but pretty soon everyone in the industry understood the potential of the simulator and this trend became general. Now every team uses one. And the graphics improved.
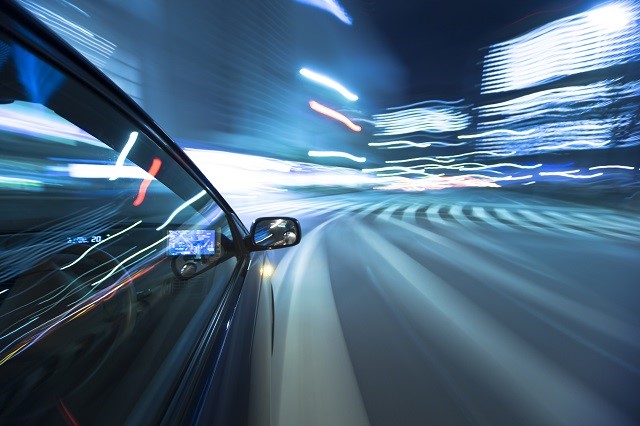
The MAT team assisted other racing sports as well. Rowing, cycling, sailing, canoeing, the bobsled, and the skeleton (the stripped-down version of the bobsled, when one rushes headfirst on an ice chute) benefited from their expertise. They chose sports where the number of variables were relatively low, but still pretty hard to take into account. For instance, their research on cycling had to combine the rigidity and mathematical simplicity of the metal frame with the “blobbiness” of the human body. For three years, the team only gathered data but, in the end, they managed to produce a mathematical equation apt enough to describe both man and machine. This produced better, lighter, and faster bikes.
Airport Efficiency
London’s Heathrow Airport also contracted the team. Since Heathrow has only two runways and traffic is very intense, they wanted to find the most efficient way of planning the coming and going of the airplanes. Using their analytics program called Race Day, MAT were able to recommend procedures based on what the users wanted to prioritize. Their solution is not to offer final solutions and take automated decisions. Instead, they make the clients aware of choices they never knew they had - and show them how those choices will affect them in time.
Big Oil
MAT developed programs for major oil and gas companies as well. They wrote software that interpreted data coming from a multitude of sensor on the drills that made their way through layers of rock and sand.
“We knew nothing about drilling. But we realized it’s not so much the drilling process as such; it’s how do you proceed when something doesn’t quite go right,”
says Caroline Hargrove, Simulation Development Engineer and later Programm Director for MAT.
Healthcare
If there’s a way to measure something, MAT will analyze it and find better alternatives on how to use it. They don’t need to intimately know the field. If they can narrow it to equations and data, they can make sense of almost everything. Healthcare is no different. Reading sensors from patients in intensive care units is just like reading the data coming from a pilot in its car.
“In sort of simplistic, nonmedical terms, if you can start to help when the body is still trying to fix it or even before the body has given up, you will be in a better position.” Peter van Manen, former head of the electronics division.
We’re really coming to terms to big data. What we can achieve with it is only up to our imaginations - and the power of our computers.
Readers also enjoyed:
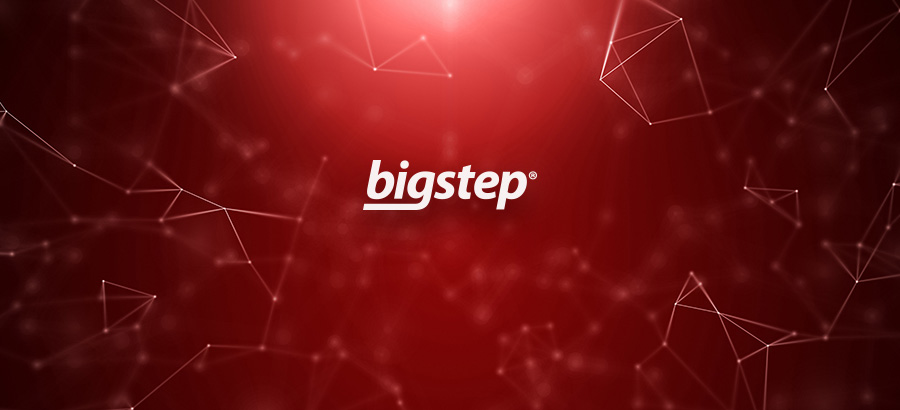
Will the cloud ever truly make its mark on the enterprise?
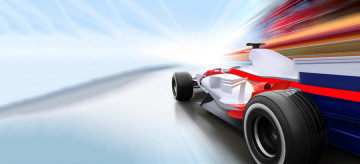
Leave a Reply
Your email address will not be published.