- Advertising
- Bare Metal
- Bare Metal Cloud
- Benchmarks
- Big Data Benchmarks
- Big Data Experts Interviews
- Big Data Technologies
- Big Data Use Cases
- Big Data Week
- Cloud
- Data Lake as a Service
- Databases
- Dedicated Servers
- Disaster Recovery
- Features
- Fun
- GoTech World
- Hadoop
- Healthcare
- Industry Standards
- Insurance
- Linux
- News
- NoSQL
- Online Retail
- People of Bigstep
- Performance for Big Data Apps
- Press
- Press Corner
- Security
- Tech Trends
- Tutorial
- What is Big Data
5 Tips & Tricks for Staying Out of the Ditch with Data Analytics
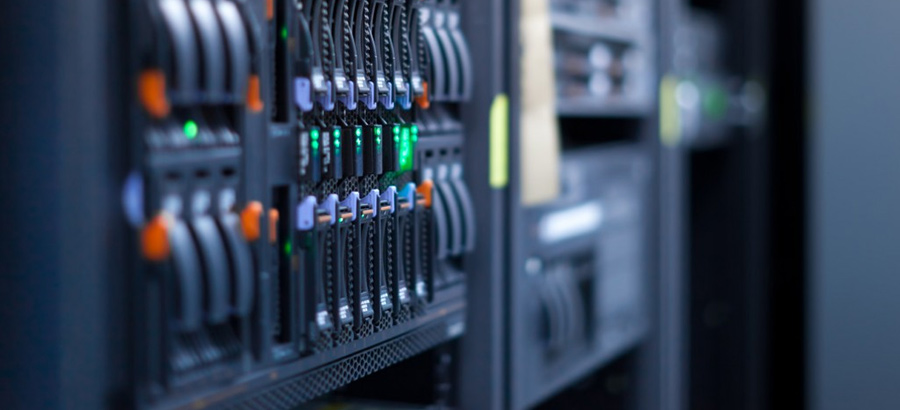
Data analytics has probably been discussed and debated as much as any of the disruptive technologies that have rocked our times. Finally, a clear set of tools, strategies, and technologies have emerged to make data analytics practical for all sizes of organizations, and the latest tools make it possible to do so without a huge team of expensive data scientists. For example, the data lake makes it possible to collect and store data before you even determine what you’ll do with it analytically. So, with all these new tools and technologies, how can you assure your data analytics won’t fall in the ditch? Here are the best tips and tricks for staying out of trouble.
1. Poor or Lack of Data Cleansing
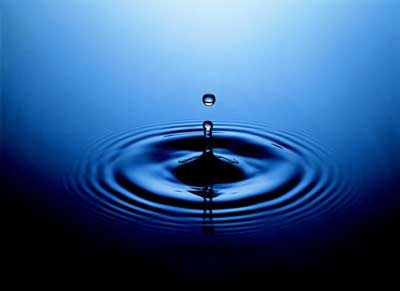
Any analytics you perform on dirty data are meaningless. Data cleansing is the hallmark of any good data analytics project. Initial cleansing must be backed by a solid data use and governance policy, so that good data stays good and all analytics performed on the data is relevant, useful, and meaningful.
2. Taking Your Bias With You Into the Analytical Process
Ah, all that data—sitting there just waiting to confirm your deeply held beliefs and assumptions. Wrong! Approaching analytics by only looking for results that confirm your hypothesis, and ignoring any results that contradict your beliefs is the best way to put a data initiative in the ditch—fast. For example, say you have a landing page that generates an impressive amount of leads. But, say that almost none of those leads were able to be qualified by sales. You can’t just take the results and call it a win. Inevitably, it was a loss. Always drop your bias at the door when approaching data analytics.
3. Being Distracted by Irrelevant Noise
Given the sheer size of data that constitutes “big data,” it is all too easy to become distracted by data sets and results that aren’t actually meaningful. For example, say your new Twitter page generates thousands of followers quickly. If few of those followers ever become customers, this is actually irrelevant noise. Keep your eyes on the road to stay out of the data ditch.
4. Making Faulty Assumptions About Correlations Versus Cause & Effect
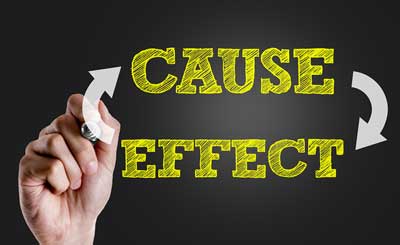
Strong correlations do not necessarily mean cause and effect, even if that seems to be a logical conclusion. For example, say that your sales take off right about the time you launch your new website, and the website has generated killer traffic. Does that mean the new website generated those new customers? Not necessarily. Examine the data until you can prove cause and effect. Never make assumptions about correlations, even when they “make sense”.
5. Using a Too-Small Sample to Achieve Statistical Significance
Big data is huge. Big data is huge like the number of Google searches conducted in a given day (3.5 billion) and the number of Facebook posts generated in a day (more than 350 million, just including those with photos). While your data sets don’t have to be quite that large to be statistically relevant, you do have to work with data sets large enough to be statistically meaningful. A few hundred transactions may or may not be enough to be relevant statistically, based on what you’re trying to find out. Always make sure your data samples are big enough to use big data analytics. You may need to switch to a tool more practical for smaller data sets.
One of the best ways to become savvy in data analytics is to see what others have done, and how they did it successfully. Visit now to see our customer stories and learn how others stayed out of the ditch—or at least got back out after a fall. Data analytics: you can do it, and Bigstep can help.
Readers also enjoyed:
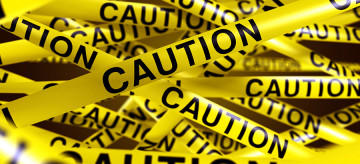
How Does Mobile and Wearable Tech Affect Your Big Data Privacy Policies?
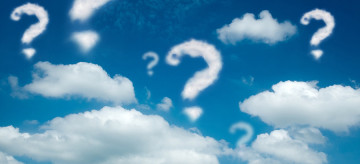
Leave a Reply
Your email address will not be published.