- Advertising
- Bare Metal
- Bare Metal Cloud
- Benchmarks
- Big Data Benchmarks
- Big Data Experts Interviews
- Big Data Technologies
- Big Data Use Cases
- Big Data Week
- Cloud
- Data Lake as a Service
- Databases
- Dedicated Servers
- Disaster Recovery
- Features
- Fun
- GoTech World
- Hadoop
- Healthcare
- Industry Standards
- Insurance
- Linux
- News
- NoSQL
- Online Retail
- People of Bigstep
- Performance for Big Data Apps
- Press
- Press Corner
- Security
- Tech Trends
- Tutorial
- What is Big Data
5 Reasons Why Your Data Analysis is Inaccurate
Are you getting inconsistent or confusing results from your big data analysis? There are several common mistakes that lead to inaccuracies. The good news is, all of these problems are relatively easy to fix (once you know what to look for), so that you can get your efforts back underway with greater success.
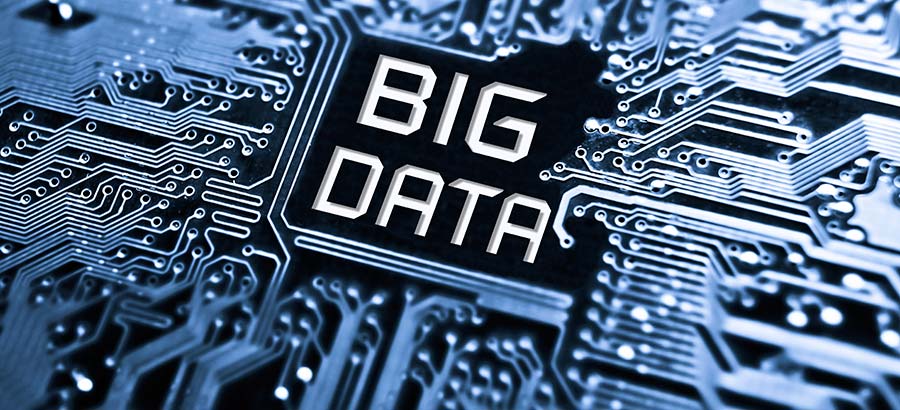
Are you getting inconsistent or confusing results from your big data analysis? There are several common mistakes that lead to inaccuracies. The good news is, all of these problems are relatively easy to fix (once you know what to look for), so that you can get your efforts back underway with greater success.
1. Your Data Isn’t Properly Cleansed
The majority of data sets hold errors like redundancies in the data (data that is entered multiple times), incomplete data, data that is outdated, or data that is simply inaccurate. Data cleansing is the process of going through the data to weed out the errors in order to assure that the remainder of the data is pure and accurate. Only by starting with good data can you render accurate analytical results.
2. You Failed to Normalize the Data
The data has to be normalized, or transferred into a consistent format in order to produce accurate results. For instance, if you run one analysis using the monthly income of your group and another analysis using their annual income, the results won’t be compatible. This sounds like a ridiculous mistake to make, but in fact, it’s more common than you’d think.
3. Your Algorithms are Inaccurate
Not all algorithms are created equally. Look at Google: they tweak their algorithms continually, always making adjustments and refinements based on what they see in real world application of the product. All data scientists should do the same. Continue to revise and refine your algorithms until you get those right. Then keep tweaking to go from better to best to perfect to even better than that.
4. Your Models are Too Complicated or Too Simplistic
Like algorithms, working the models out correctly takes time and some trial and error. It’s extremely easy to develop models that are entirely too complex, and just as easy to come up with models that are far too simplistic. If the data analytics is not yielding the results that you think make sense, take a look at your models. See if simplifying the model or perhaps asking a little more out of the data might help you get the analytics just right.
5. You are Holding a Bias That Skews Your Interpretations
Of all the problems on this list, this is the most difficult to identify and even harder to correct. Every researcher wants to believe that they are 100 percent objective. Yet few if any truly are. What biases are you holding that might be causing your interpretations of what the data is telling you to be off? It’s helpful to get a second (or even third, fourth, and fifth) pair of eyes on the data and analytical results to see if you are actually approaching your interpretations with the objectivity a scientist needs to have.
With some effort, your data analytics will render accurate and reliable results in no time.
Readers also enjoyed:
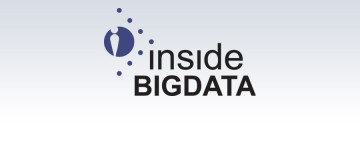
Expert Interview with Daniel D. Gutierrez on Big Data Headlines
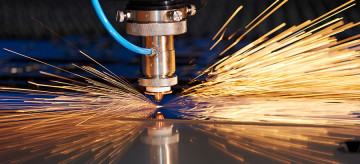
Leave a Reply
Your email address will not be published.