- Advertising
- Bare Metal
- Bare Metal Cloud
- Benchmarks
- Big Data Benchmarks
- Big Data Experts Interviews
- Big Data Technologies
- Big Data Use Cases
- Big Data Week
- Cloud
- Data Lake as a Service
- Databases
- Dedicated Servers
- Disaster Recovery
- Features
- Fun
- GoTech World
- Hadoop
- Healthcare
- Industry Standards
- Insurance
- Linux
- News
- NoSQL
- Online Retail
- People of Bigstep
- Performance for Big Data Apps
- Press
- Press Corner
- Security
- Tech Trends
- Tutorial
- What is Big Data
When Data Meets Philosophy
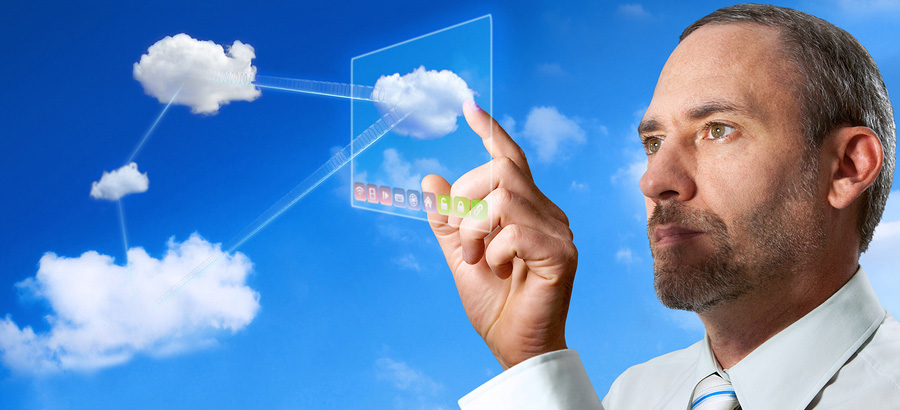
When it comes to big data, philosophy is a bit overwhelmed. Attitudes vary tremendously. Some people fear it, others see it as necessary (but distrust it), others warmly embrace it - yet caution that we need new ethical mechanisms to keep it in check, while others believe we need not concern ourselves with ”why” something happens, and instead just let the data ”speak for itself”.
The common theme among these philosophers is that
- there is something called big data
- we don’t understand it very well
- we generate it whether we want to or not
- it’s here to stay
- what shall we do about it?
Since big data represent such a fundamental shift in our lives, definitions also vary. For Rob Kitchin, a big database does not necessarily mean big data. Instead, what qualifies as big data is.
- huge in volume, consisting of terrabytes or petabytes of data;
- high in velocity, being created in or near real-time;
- diverse in variety in type, being structured and unstructured in nature, and often temporally and spatially referenced.
- exhaustive in scope, striving to capture entire populations or systems (n=all)
- fine-grained in resolution, aiming to be as detailed as possible, and uniquely indexical in identification;
- relational in nature, containing common fields that enable the conjoining of different datasets;
- flexible, holding the traits of extensionality (can add new fields easily) and scalable (can expand in size rapidly).
The sheer scale of the concept means that, for now, we lack the means for creating a meaningful relationship with it. In the words of Melanie Swan,
“[…] the cornerstone issue is an incomplete subjectivation by both sides; neither party, data nor humans, has a complete and full understanding (humans) or representational model (data) of the other.”
It’s relatively easy to grasp why we, as humans, have some difficulty relating to the concepts of big data, but how can this “representational model” have any idea of us? Perhaps the only easy answer is that we are too human and computers are too… well, computational. How can a program understand something about a person that clicks around a website? The program simply builds a “heat map” and then, using predefined algorithms, makes assumptions about that person and interprets the user’s intentions. This is how such a program “sees” us. On the other hand, a human has a hard time accepting the idea that every cursor move and every keyboard stroke are recorded and interpreted in a specific context. Maybe in a high-tech future, where human-computer hybridization is common, this relationship will become clearer. For not, we can only observe its shortcomings and try to correct them.
And there’s the correlation-causation problem. Schonberger and Cukier write in their 2013 book Big Data: “Correlations let us analyze a phenomenon not by shedding light on its inner workings but by identifying a useful proxy for it. […]”
“With correlations, there is no certainty, only probability. But if a correlation is strong, the likelihood of a link is high.”
Computers have become pretty good at recommending books, movies and music. They do this by matching metadata and a variety of parameters, not by judging the inner qualities of the work in question. That is, they use probability and not certainty. However, if the number of data points is high enough, those “mindless” correlations will get pretty close to a fine critic’s recommendations. That works well enough for most people, but Andrej Zwitter thinks that we are more vulnerable if we are to “believe what we see without knowing the underlying whys”.
Besides commercial ventures, who will most likely benefit from the big data revolution? Zwitter believes that there are three categories of people most likely to understand and put big data to use:
The first category is that of the political campaign observers, think tank researchers, and other investigators who use big data as a natural extension of their work. They will attempt to adapt to and, if need be, change public opinion to suit their goals.
The second category is that of law enforcement and social services. They will need to re-conceptualize individual guilt, probability and crime prevention.
Finally, the third category belongs to the states who will progressively redesign the way they develop their global strategies based on global data and algorithms rather than regional experts and judgment calls.
Corporations and governments have traditionally used big data, but isn’t it conceivable that, with the appearance of WikiLeaks and the change of perception it has brought us, we’ll see another category emerge - that of the “anonymous public”? And who’s to say it won’t be just as powerful as the other ones?
Readers also enjoyed:
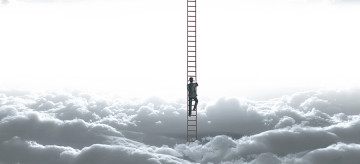
Why Big Data will Always Need Gut Instincts Too
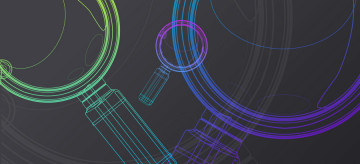
Leave a Reply
Your email address will not be published.