- Advertising
- Bare Metal
- Bare Metal Cloud
- Benchmarks
- Big Data Benchmarks
- Big Data Experts Interviews
- Big Data Technologies
- Big Data Use Cases
- Big Data Week
- Cloud
- Data Lake as a Service
- Databases
- Dedicated Servers
- Disaster Recovery
- Features
- Fun
- GoTech World
- Hadoop
- Healthcare
- Industry Standards
- Insurance
- Linux
- News
- NoSQL
- Online Retail
- People of Bigstep
- Performance for Big Data Apps
- Press
- Press Corner
- Security
- Tech Trends
- Tutorial
- What is Big Data
Robots to Judge and Hire Us
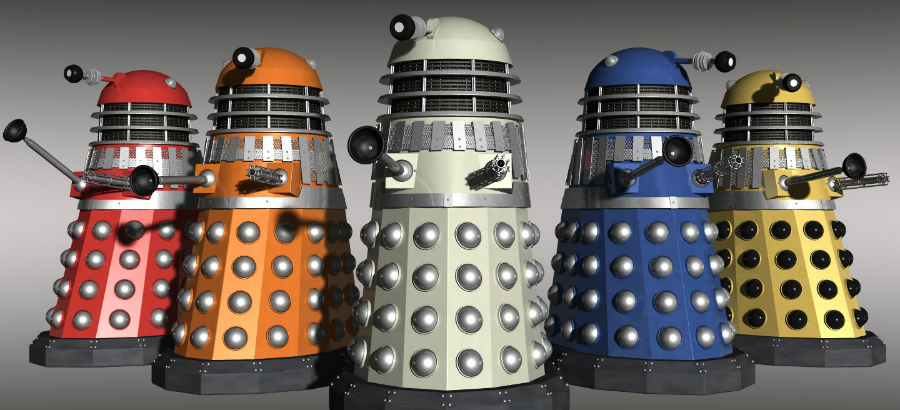
The New York Times says artificial intelligence can hire people and do a pretty good job at it. The Wall Street Journal says the same. Some are not convinced. The story of predictive software is trickier than it seems.
In this article, Kaiser Fung and Andrew Gelman analyze the claim that HR software such as Gild, Entelo, Textio, Doxa, GapJumpers and others can offer a bias-free hiring environment. While nobody goes out of their way in thinking that the hiring process can be completely automated, there is the general feeling that machines can provide a more objective approach than humans when it comes to hiring. Analytics and AI could be deployed to identify the right people for the right job far more efficiently than humans can.
“Readers should be made aware that these HR problems are highly complex, administrative data are fraught with defects, and the outcomes are difficult to quantify.”
Not so, say the two Daily Beast authors. They point out a bunch of false premises, “dubious claims” and quite a bit of confusion favoring the HR algorithms over the people.
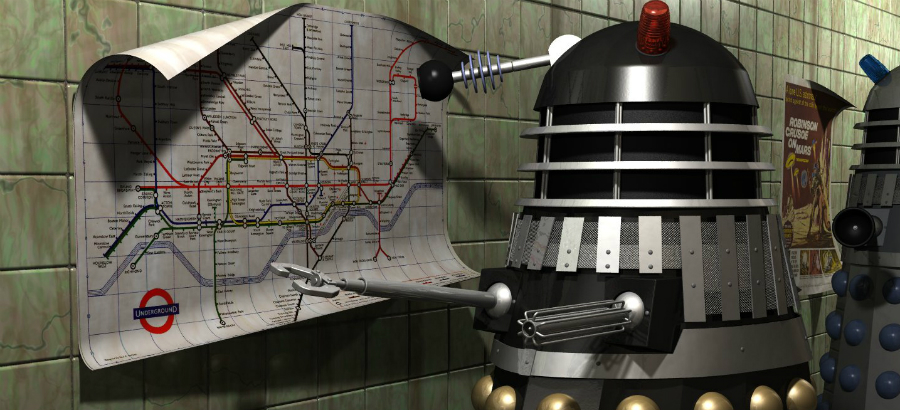
The biggest problem seems to be that nobody has any precise idea about what the objective of automated hiring is. Is it about predicting job performance or is it about seeking the best people? Is it to offer a more diverse workforce or is it about predicting the likelihood that someone would be interested in a job? These are all matters that the Times article refers to without realizing that each of those questions must rely on unique statistical models. Also, in the case of predicting job performance, the complexity of such a task is increased by other unmentioned factors, such as experience, quality of management and performance of team members.
Another worthwhile (and perhaps shocking) idea is that, according to the Daily Beast, there is no machine free of human biases. The models that are fed into the AI are all based on previous generations of data, gathered when there were only human operators (and thus affected by racial or gender biases). This means that the predictive models to be constructed are themselves biased.
“If these employers never had diverse applicants, or never made many minority hires, there is scant data available to create a predictive model that can increase diversity!”
The difficult task of quantifying is that we don’t always know what to quantify. Let’s say the HR software predicts that an employee is likely to quit within a year unless the manager intervenes. The manager, prompted by the program, steps in and addresses the issue. The employee does not resign within the year. Did this mean that the intervention worked or that the software made a false prediction?
The same problem is noticed by Katherin Noyes at CIO.com:
“Such data, however voluminous, still won’t necessarily give you the “why?” part of the equation.”
But qualitative data analysis is beginning to change. Stepping away from the human resources field, we can find clever tools like YouEye, a software able to translate visual interaction into meaningful information that can be used to “track user sentiment”.
“the researcher typically assigns a positive or negative value to the sentiment being expressed in the qualitative data, and then assigns a numerical value to the strength of that sentiment”
But while the tools are slowly getting more sophisticated, it’s nevertheless more accurate to predict something with less “shades of meaning” rather than with more. There are a lot of useful things to be learned from the “quant” and “qual” approach, but for the time being it’s an ongoing challenge. Like any new technology, it needs to be handled with care and allowed to mature. Reaching too many (or too few) conclusions too soon will almost certainly lead to failure.
Wouldn’t you say so? Your comments are welcome.
Leave a Reply
Your email address will not be published.