- Advertising
- Bare Metal
- Bare Metal Cloud
- Benchmarks
- Big Data Benchmarks
- Big Data Experts Interviews
- Big Data Technologies
- Big Data Use Cases
- Big Data Week
- Cloud
- Data Lake as a Service
- Databases
- Dedicated Servers
- Disaster Recovery
- Features
- Fun
- GoTech World
- Hadoop
- Healthcare
- Industry Standards
- Insurance
- Linux
- News
- NoSQL
- Online Retail
- People of Bigstep
- Performance for Big Data Apps
- Press
- Press Corner
- Security
- Tech Trends
- Tutorial
- What is Big Data
How to Run a Big Data Benchmarking Test
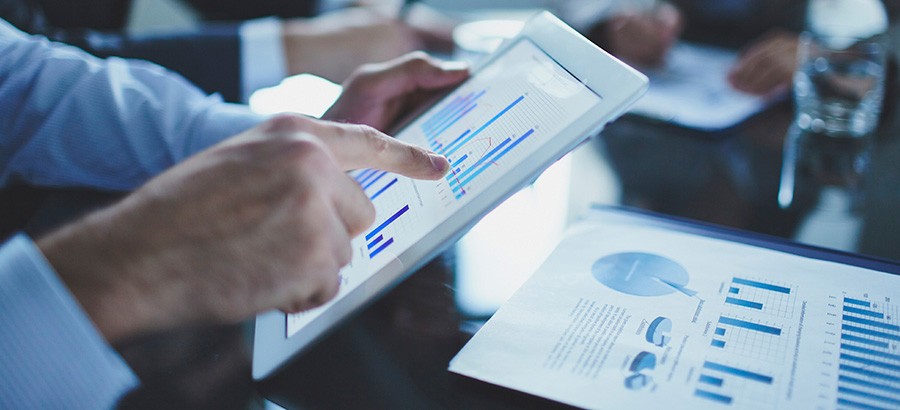
Are you preparing to conduct benchmark testing on your big data operations? This testing is essential to determine whether your efforts and changes are moving in the right direction or the wrong direction. But your testing, if not done properly, can lead you even further off the path. Here is how to conduct a big data benchmarking test that will give you accurate, true results so you will know where to head from here.
Be Sure That the Test is Done at the Right Level and Scale
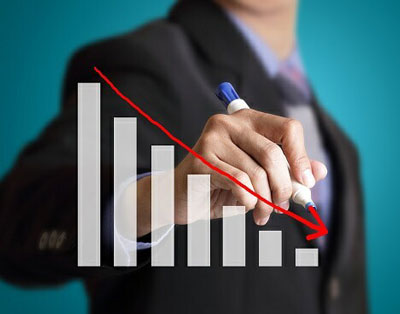
What scale are you testing on? You can test at the micro level, which is testing at the lowest level of systems operations. You can test at the functional or component level, which is testing of a pre-determined high level function. Or, you can test at the application level, which is testing of the performance of a specific scenario of an application. Also, be sure you are testing at the correct data scale, scale of concurrency (number of jobs and number of tasks within each job), cluster scale (number of notes or racks), and node scale (per node hardware size). If the level and scale of testing is not commensurate, your testing won’t yield accurate results. It’s like comparing apples to oranges.
Be Sure You Understand (About) What the Performance Level Should Be
What is your best educated guess as to what the performance should be? If the test indicates results wildly off base from what you expected, you need to make sure something isn’t off kilter. If the results don’t look accurate, check again to make sure your tests were at the right level and scale, and that you’re comparing ‘apples to apples’. For example, if you expect performance to improve threefold, but the testing indicates performance has improved a thousand fold, the test is probably not accurate. Make sure the results make sense.
Be Sure That Benchmarks Aren’t Inherently Biased
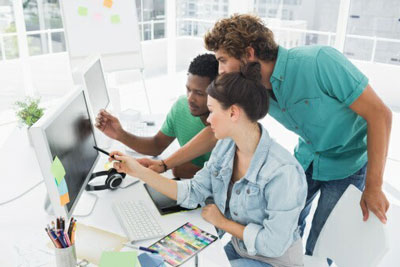
Did you (or someone else) deliberately or accidentally select a workload, equipment, or settings that could skew your results? Was the job hand-picked to be easier to perform, thereby making the results look far better than they would if a more random or average workload was chosen? Be sure to test fairly, or results could be out of proportion based on something entirely different than what you were actually benchmarking for.
Communicate the Results the Right Way
After you’ve taken precautions to assure that the tests compared like things, were set up fairly, and weren’t biased by outside circumstances, you need to be sure that the results are communicated to stakeholders accurately. For example, don’t try to shorten a 42 percent improvement by stating it as 50 percent improved. Be as accurate as possible when communicating the results so that everyone knows exactly what (if any) performance improvements there were and can make solid decisions based on the information provided.
When it comes to big data, your benchmarking tests will prove that there’s no faster, more powerful tool around than the Full Metal Cloud by Bigstep. Visit today to see what the Full Metal Cloud can do for your big data performance.
Readers also enjoyed:
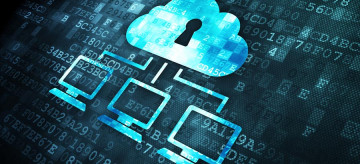
How Can You Be Sure Your Information is Secure in the Cloud?
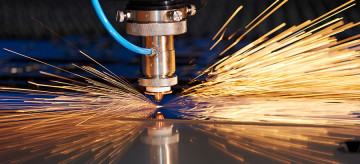
Leave a Reply
Your email address will not be published.